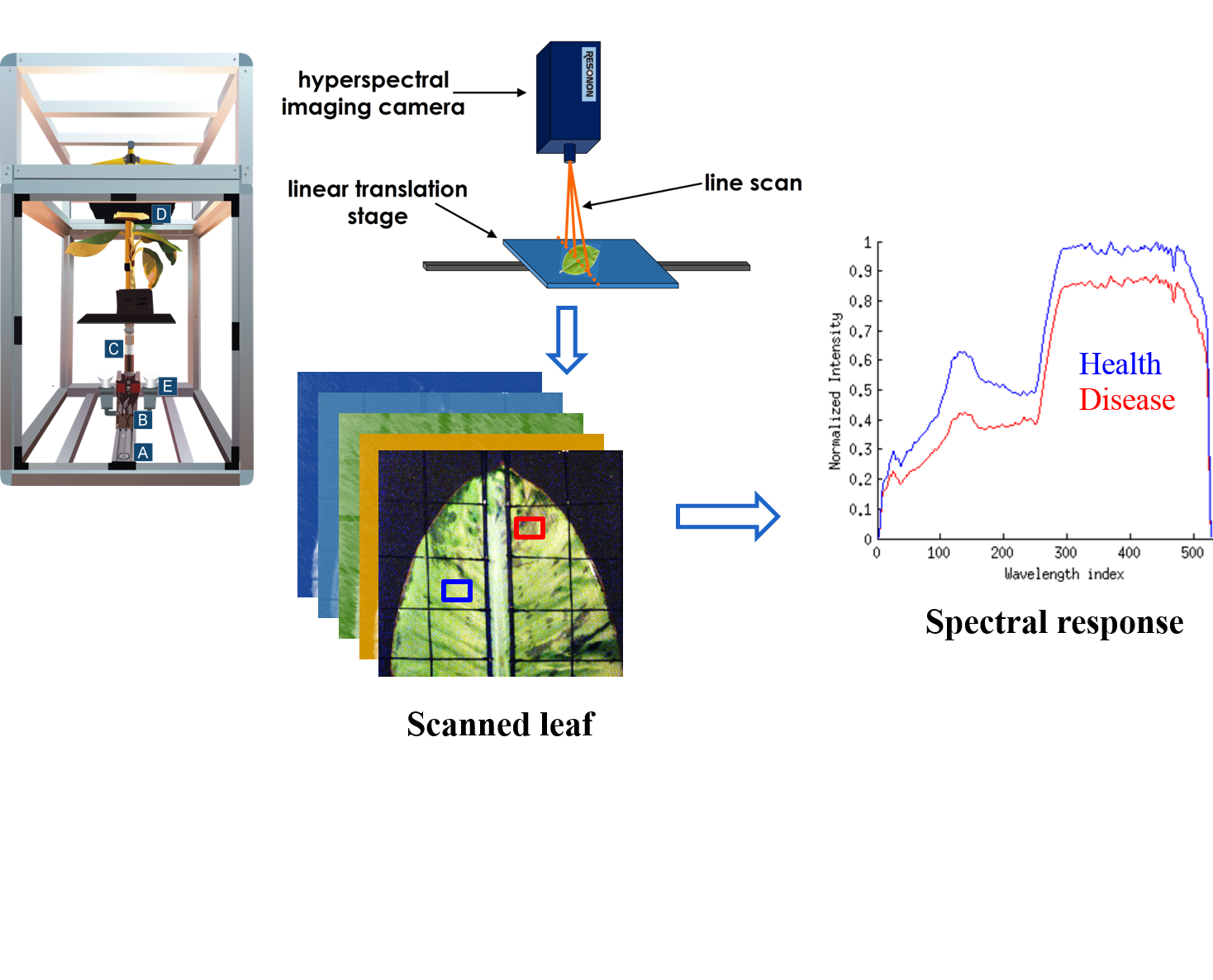
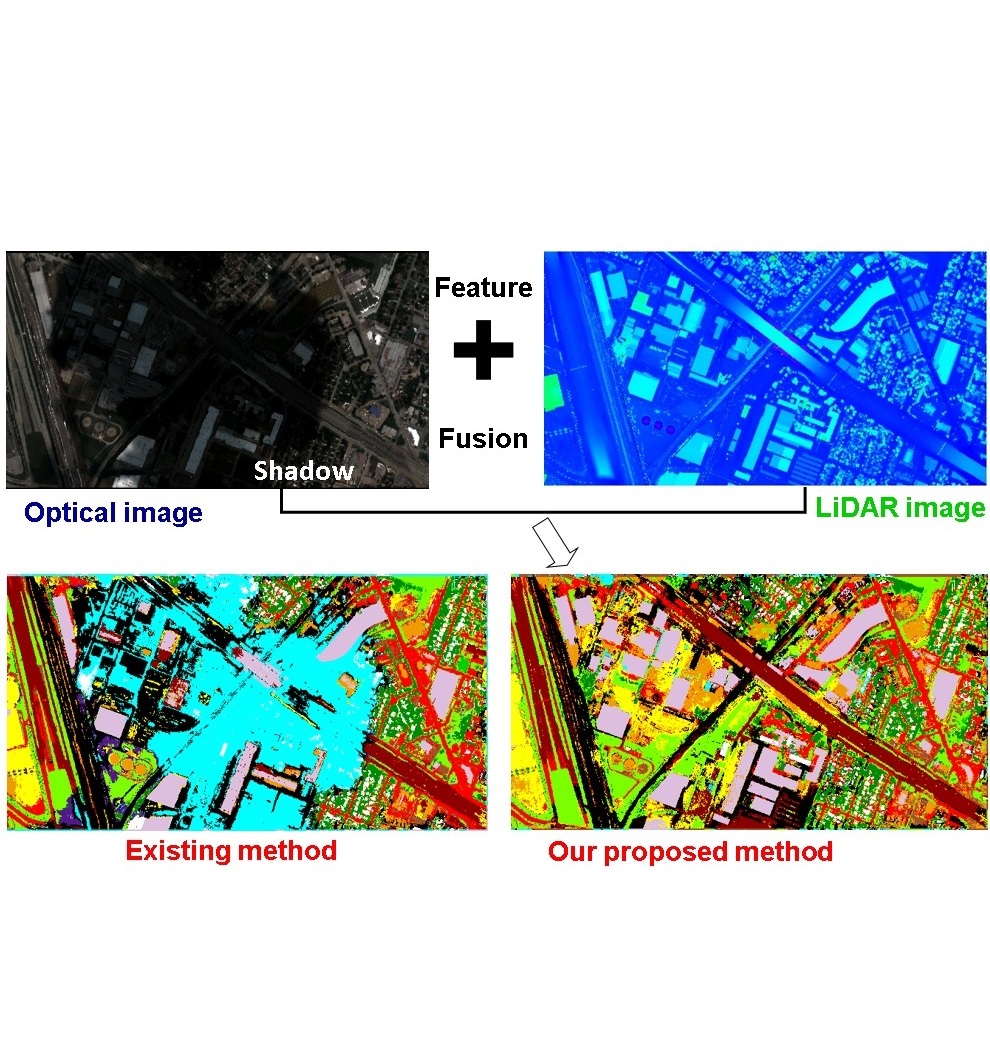
Nowadays, compact and low-cost hyperspectral cameras are being developed that can be applied in close-range settings, such as unmanned aerial vehicles, with cameras mounted on agricultural equipment or conveyer belts, in lab environments and even in handheld modus. The close-range setting allows for flexible acquisition and generates hyperspectral imagery of high spatial/spectral/temporal resolutions, leading to much finer material characterization (e.g., plant disease detection, food inspection, etc.) than was possible before.
We developed novel methods for close range hyperspectral image (including visible and near infrared spectrum) processing and its applications to detect plant disease and fruit bruise.
Nowadays, advanced technology in remote sensing allows us to get multi-sensor and multi-resolution data from the same region. However, fusion of these data sources for classification remains challenging
problems.
We proposed a graph-based fusion method to couple dimensionality reduction and data fusion of multi-sensor remote sensing data. Our approach by fusing hyperspectral and LiDAR data for classification won the "Best Paper Challenge" Award of the 2013 IEEE GRSS Data Fusion Contest. (PDF)
Despite advances in sensor technology, hyperspectral (HS) images are inevitably degraded by noise and blur, which can affect information retrieval and content interpretation. Using denoising
and deblurring as a preprocessing tool will improve various post-processing tasks, e.g. classification, target detection, unmixing, etc.
We propose a novel restoration algorithm for HS images. Our method first uses PCA to decorrelate the HS images and separate the information content from the noise. The first k PCA channels contain most information of the HS image, and the remaining B − k PCA channels (where B is the number of spectral bands of HS image) mainly contain noise. If deblurring is performed on these noisy and high-dimensional
B − k PCs, then it will amplify the noise of the data cube and cause high computational cost in processing the data, which is
undesirable. Therefore, we only restored the first k PCA channels. We remove the noise (without restoration) in the remaining PCA channels using a soft-thresholding scheme.(New)
Recent advances in remote sensing technology have led to an increased availability of a multitude of satellite and airborne data sources, with increasing spatial, spectral and temporal resolutions. Additionally, at lower altitudes, airplanes and Unmanned Aerial Vehicles (UAVs) can deliver very high resolution data from targeted locations. Remote sensing images of very high geometrical resolution can provide a precise and detailed representation of the surveyed scene. Thus, the spatial information contained in these images is fundamental for any application requiring the analysis of the image. (PDF)
Hyperspectral sensors collect information as a set of images represented by hundreds of spectral bands. While offering much richer spectral information than regular RGB and multispectral images for classification, this large number of spectral bands creates also a challenge for traditional spectral data processing techniques. A new framework of semi-supervised feature extraction method was proposed to reduce the dimension of both the original hyperspectral data. Our technique can use limited labeled samples to improve the classification results (PDF).